The following describes some of the current projects being conducted in the ALC lab...
​
Pre-stimulus EEG phase predicts auditory performance
There is increasing evidence from neurophysiological recordings that ongoing oscillatory brain states shape perception, cognition, and performance (for review, see Addante et al., 2011; Buzsáki, 2006, pp. 263-333; Jenson & Mazaheri, 2010; VanRullen, 2011; 2016a). For example, Busch et al. (2009) found that the pre-stimulus phases of EEG oscillations in the high theta/alpha range (~6-12 Hz) differed between hit and miss trials in a visual detection task, with a monotonically decreasing trend in accuracy from an individual’s most accurate phase to ~180° opposite. There are comparably fewer studies in the auditory domain, and results have been mixed (for review, see Zoefel & VanRullen, 2016). In regard to phase, several have shown that phase predicts auditory detection when oscillations are entrained by background sound with inherent rhythmicity (Henry & Obleser, 2012; Ng et al., 2012). However, a relationship between pre-stimulus phase and accuracy without entrainment or background sounds has so far been difficult to demonstrate.
​
In a series of recent experiments conducted in collaboration with the 711th Human Performance Wing of the U.S. Air Force Research Laboratory and the Human Neuroscience and Visual Cognition Lab at Wright-State University, we are investigating the relationships between spontaneous pre-stimulus EEG phase and accuracy in auditory tasks. Figure 1 depicts the task from one of these experiments. Listeners heard sequences of tones which could be either "high" or "low" in frequency and had to identify the sequence (High-Low-High, Low-High-Low, High-Low-Low, etc.). We compared correct to incorrect trials in regards to EEG phase just prior to the onset of tone sequences.
​
​

Figure 1. Depiction of the task used in the first experiment. The onset of the tone sequence is represented by the vertical dashed line. Response options, a single-trial EEG trace, and 10 Hz EEG phase are shown for reference. Of interest to use was whether or not the phase of EEG just prior to stimulus onset predicted performance (i.e., whether or not an individual would be correct or wrong on that trial).
Figure 2 shows the relationship of pre-stimulus EEG phase to correctness in two different ways. For Figure 2a (top panel), a phase-opposition sum (POS) measure was computed based on inter-trial phase consistency (ITPC). Generally, "hotter" colors in the figure indicate larger phase differences between correct and incorrect trials. For further review of this measure, see VanRullen (2016). In our data strong and significant phase opposition was observed between ~6-11 Hz from ~200 - 100 ms prior to stimulus onset. Examination of individual data (lower panel) showed that this trend was consistent across individuals (thin black lines).
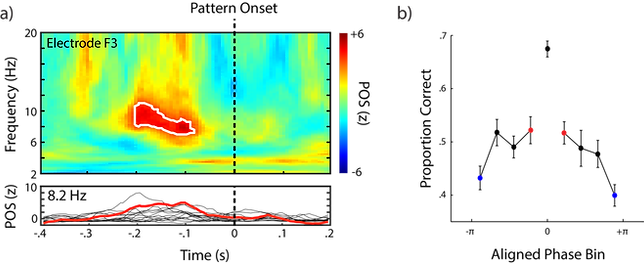
Figure 2. (a) The top panel shows POS for a range of time-frequency points at a left frontal electrode (F3). The white outline highlights a region of significance after correcting for multiple comparisons (channels and time-frequency points using fdr). The bottom panel shows individual POS across time at 8.2 Hz. The red line shows POSz after combining across participants (using Rayleigh's z; cf. VanRullen, 2016). (b) Mean proportion correct binning trials based on phase and aligning individuals according to the best performing phase bin. Error bars represent within-subject standard errors of the mean.
When individual trials were broken into 9 different bins based on phase at 8.2 Hz and -180 ms relative to pattern onset. Proportion correct was computed for each bin. Individual data was then aligned such that an individuals' most accurate bin was a 0 radians (see Figure 2b). Mean proportion correct across the other bins yielded a clear relationship with pre-stimulus phase such that worst performance was at the opposite phases (i.e., -/+ pi).
In follow-up experiments, we are asking: 1) Can listeners learn to optimally phase align pre-stimulus oscillations if stimulus onsets are known? and 2) How does task complexity impact the predictive capability of pre-stimulus phase?
​
Effects of training regimen on auditory learning and generalization
Not all training regimens produce the same learning outcomes. This interests us for two reasons. First, if auditory training is to be used for rehabilitative purposes, it would be useful to know what training regimens produce the best results. Second, the processes involved in auditory learning can potentially be gleamed from studies that compare training regimens. For instance, theories that posit learning results from focusing attention on the discrimination relevant dimensions can in some circumstances predict different outcomes from theories that posit learning results from long-term changes stimulus representations.
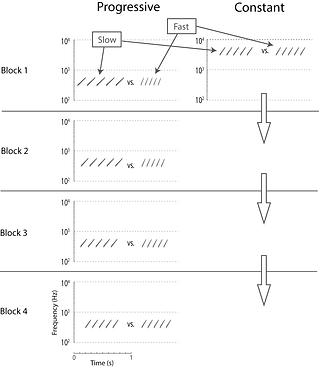
Figure 3. Depiction of the task used in Wisniewski, Radell, Church, & Mercado (2017). In this example, the low frequency range starts with an easy "Fast" vs. "Slow" contrast that progressively gets harder over the course of blocks. The high frequency range remains constantly difficult throughout training.
In a number of studies conducted in collaboration with the Neural and Cognitive Plasticity lab at SUNY Buffalo and the SUCCESS lab at Georgia State University, we have compared learning outcomes after training that progresses from easy-to-hard with training that remains hard throughout. Figure 3 depicts an example of the methods used in one experiment (see Wisniewski et al., 2017 – PloS ONE). Participants were trained to discriminate trains of frequency-modulated (FM) tonal sweeps based on their rate. In one frequency range (300 – 600 Hz) the contrast between “Fast” and “Slow” rates progressed from easy-to-hard over the course of training blocks (Progressive Condition). In the other frequency range (3000 – 6000 Hz), the “Fast” vs. “Easy” contrast was hard for every training block (Constant Condition). Assignments of conditions to frequency ranges were counterbalanced across participants. After training, listeners were tested on their ability to discriminate rates of FM sweep trains in both frequency ranges.
​
Figure 4 shows discrimination accuracy (A’) in a post-training test. Accuracy was higher for the frequency range trained in a progressive manner compared to the frequency range trained in the constant manner. Though attentional spotlighting theories can explain a number of learning phenomena, they have a difficult time explaining this result. The relevant dimension (FM rate) was the same for both frequency ranges, yet a progressive advantage was still seen within-subjects. In contrast, simulations we've conducted with a connectionist model of representational plasticity (for model details, see Wisniewski, 2017 - Experimental Brain Research) have been able to reproduce data trends. We are extensively exploring the impacts of stimulus sequencing on learning in trained tasks as well as how it generalizes to untrained tasks (e.g., from FM rate discrimination, to frequency range discrimination). We are also exploring how sequencing may effect learning that occurs when individuals are simply exposed to sounds without any associated task.
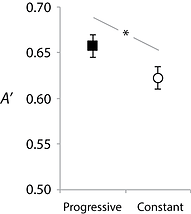
Figure 4. "Fast" vs. "Slow" discrimination accuracy as reflected in the A' signal detection measure. Error bars show within-subject standard error of the mean. The asterisk marks significance (p<.05).
Exploring neural correlates of metacognitive judgments
Metacognitive monitoring of performance is important for decision making. For example, if a person is overconfident in his/her ability to listen and accurately understand a conversation in a loud room, they might not ask for clarification when necessary. This is especially critical to those working in risky environments, such as a war zone. For example, it is critical that an officer with wounded soldiers can accurately detect the sound of a rescue helicopter. If s/he is over- or under- confident in the identification of an incoming air craft, inappropriate actions could lead to disastrous consequences.
Most EEG studies examining metacognitive judgments (e.g., confidence, judgments-of-learning, feelings-of-knowing, etc.) have been conducted in the visual domain. Additionally, researchers often study metacognition using higher-level cognitive tasks, such as recognition of studied words or pictures. However, it is also important to understand neural activity related to metacognition in the auditory domain, using tasks that do not require semantic comprehension or memorization.
Recently, we have used the event-related potential (ERP) to study the stages of processing related to metacognitive judgments, specifically, confidence. In an auditory detection task, we asked participant to listen for a tone embedded in white noise. Following each response, they made confidence ratings about the accuracy of their response. This work revealed larger tone-locked N1, P2, and P3 amplitudes on trials for which participants rate their response accuracy with high confidence compared to low confidence. Figure 5 illustrates these differences. We suggest that metacognitive judgments can track both sensory- and decision-related processes (indexed by the N1 and P3, respectively).

Figure 5. Adapted from Zakrzewski, Wisniewski, Iyer, and Simpson (2019). Scalp maps of N1, P2, and P3 broken up by confidence level.
Future work will examine not only the neural correlates of metacognitive judgments, such as confidence, but also how EEG can be used to study metacognitive accuracy (how well metacognitive judgments match performance accuracy on a trial-by-trial basis). Do those with high metacognitive accuracy show different EEG/ERP activity compared to those with low metacognitive accuracy?
​
Relatedly, how does training and subsequent learning affect metacognitive abilities? Also, can metacognitive ability transfer across stimuli, tasks, and sensory domains? These questions are related to a project described above: Effects of training regimen on auditory learning and generalization. Many studies looking at metacognitive accuracy hold participants’ performance constant, at their ~71% correct threshold. However, maintaining task performance eliminates the opportunity to look at ways in which performance and metacognitive accuracy are related. One goal of the ALC lab is to ask whether metacognitive accuracy tracks perceptual sensitivity if perceptual sensitivity is manipulated through training regimens.
Oscillatory Dynamics of EEG associated with Effortful Listening
Many individuals report that listening is very effortful, even though they achieve levels of listening accuracy comparable to those reporting little effort. By consequence, these individuals choose to avoid situations wherein listening effort is likely to be high, or they suffer from increased fatigue following situations that require extensive effort. This remains a major problem for people with hearing loss and other auditory-related disorders. Our group, in collaboration with the 711th Human Performance Wing of the U.S. Air Force Research Laboratory, has extensively explored how oscillatory dynamics of EEG relate to this “listening effort” (see Wisniewski, 2017 – Ear & Hearing; Wisniewski et al., 2015 – NeuroReport; Wisniewski, Thompson, & Iyer, 2017 – Psychophysiology; Wisniewski, Iyer, Thompson, & Simpson, 2018 – Hearing Research). The goals of this research are to: 1) identify objective indices of “listening effort” to complement subjective self-report measures (e.g., ratings of effort), 2) characterize the involvement of the specific cognitive processes in effortful listening, and 3) evaluate methods for reducing effort.
​
Data from our 2017 Psychophysiology paper is presented below for a introduction to this project. Figure 6a depicts the auditory delayed match-to-sample task that was employed. On each trial, listeners heard two FM sweep sounds presented back-to-back (sound A, then sound B). There was then a 2.5 s retention interval, after which a comparison sound was presented (sound X). Listeners' task was to indicate whether sound X matched A or B. There were "easy", "difficult", and "impossible" conditions. In the "easy" condition A and B differed well above listeners' discrimination threshold. In the "difficult" condition, they differed at participants ~71% correct threshold. Unbeknownst to the listeners, the "impossible" condition contained identical sounds for A, B, and X. Figure 6b shows the event-related spectral perturbation (ERSP) at electrode Fz averaged across all three difficulty conditions. Both theta (~4-8 Hz) and alpha (~8-13 Hz) power increases during the retention interval. More importantly, Figures 6c and 5d show that these power enhancements are strongest for the "difficult" condition. The "easy" and "impossible" conditions show relatively weak enhancements. The demonstration of this non-monotonic trend across levels of task difficult is important because it demonstrates a similarity between power enhancements and other measures of mental effort in non-auditory works (for review, see Richter, 2016). Other works we have published have ruled out explanations that acoustics (Wisniewski et al., 2015 - NeuroReport) and/or task accuracy (Wisniewski et al., 2018 - Hearing Research) drive these effects.

Figure 6. (a) Depiction of the task used in Wisniewski et al.(2017). (b) ERSP averaged across all task difficulty conditions. "Hot" colors indicate enhancement of power relative to baseline. "Cool" colors indicate suppression. (c) Traces of theta power (4-8 Hz) and (d) alpha power (8-13 Hz) for each of the difficulty conditions.
In many studies we conduct in the ALC laboratory we take advantage of independent components analysis (ICA). ICA identifies temporally independent components (ICs) in EEG data. Each IC has a fixed scalp projections and many studies have shown that these scalp projections are well accounted for by single equivalent current dipoles in the brain. Like we can analyze the ERSP for any one channel, we can analyze the ERSP for any one IC. For an excellent review on ICA, see Arnaud Delorme's website.